Pandas Cheat Sheet
Contents
Pandas Cheat Sheet#
Setup Environment#
# ensures you are running the pip version associated with the current Python kernel
import sys
!{sys.executable} -m pip install requests
!{sys.executable} -m pip install scipy
!{sys.executable} -m pip install matplotlib
!{sys.executable} -m pip install scikit-learn
!{sys.executable} -m pip install plotly
Requirement already satisfied: requests in c:\users\wsaye\pycharmprojects\wmsayer.github.io\venv\lib\site-packages (2.28.1)
Requirement already satisfied: charset-normalizer<3,>=2 in c:\users\wsaye\pycharmprojects\wmsayer.github.io\venv\lib\site-packages (from requests) (2.1.1)
Requirement already satisfied: certifi>=2017.4.17 in c:\users\wsaye\pycharmprojects\wmsayer.github.io\venv\lib\site-packages (from requests) (2022.12.7)
Requirement already satisfied: urllib3<1.27,>=1.21.1 in c:\users\wsaye\pycharmprojects\wmsayer.github.io\venv\lib\site-packages (from requests) (1.26.13)
Requirement already satisfied: idna<4,>=2.5 in c:\users\wsaye\pycharmprojects\wmsayer.github.io\venv\lib\site-packages (from requests) (3.4)
Requirement already satisfied: scipy in c:\users\wsaye\pycharmprojects\wmsayer.github.io\venv\lib\site-packages (1.9.3)
Requirement already satisfied: numpy<1.26.0,>=1.18.5 in c:\users\wsaye\pycharmprojects\wmsayer.github.io\venv\lib\site-packages (from scipy) (1.23.5)
Requirement already satisfied: matplotlib in c:\users\wsaye\pycharmprojects\wmsayer.github.io\venv\lib\site-packages (3.6.2)
Requirement already satisfied: kiwisolver>=1.0.1 in c:\users\wsaye\pycharmprojects\wmsayer.github.io\venv\lib\site-packages (from matplotlib) (1.4.4)
Requirement already satisfied: cycler>=0.10 in c:\users\wsaye\pycharmprojects\wmsayer.github.io\venv\lib\site-packages (from matplotlib) (0.11.0)
Requirement already satisfied: pyparsing>=2.2.1 in c:\users\wsaye\pycharmprojects\wmsayer.github.io\venv\lib\site-packages (from matplotlib) (3.0.9)
Requirement already satisfied: numpy>=1.19 in c:\users\wsaye\pycharmprojects\wmsayer.github.io\venv\lib\site-packages (from matplotlib) (1.23.5)
Requirement already satisfied: packaging>=20.0 in c:\users\wsaye\pycharmprojects\wmsayer.github.io\venv\lib\site-packages (from matplotlib) (22.0)
Requirement already satisfied: fonttools>=4.22.0 in c:\users\wsaye\pycharmprojects\wmsayer.github.io\venv\lib\site-packages (from matplotlib) (4.38.0)
Requirement already satisfied: python-dateutil>=2.7 in c:\users\wsaye\pycharmprojects\wmsayer.github.io\venv\lib\site-packages (from matplotlib) (2.8.2)
Requirement already satisfied: pillow>=6.2.0 in c:\users\wsaye\pycharmprojects\wmsayer.github.io\venv\lib\site-packages (from matplotlib) (9.3.0)
Requirement already satisfied: contourpy>=1.0.1 in c:\users\wsaye\pycharmprojects\wmsayer.github.io\venv\lib\site-packages (from matplotlib) (1.0.6)
Requirement already satisfied: six>=1.5 in c:\users\wsaye\pycharmprojects\wmsayer.github.io\venv\lib\site-packages (from python-dateutil>=2.7->matplotlib) (1.16.0)
Requirement already satisfied: scikit-learn in c:\users\wsaye\pycharmprojects\wmsayer.github.io\venv\lib\site-packages (1.2.0)
Requirement already satisfied: threadpoolctl>=2.0.0 in c:\users\wsaye\pycharmprojects\wmsayer.github.io\venv\lib\site-packages (from scikit-learn) (3.1.0)
Requirement already satisfied: numpy>=1.17.3 in c:\users\wsaye\pycharmprojects\wmsayer.github.io\venv\lib\site-packages (from scikit-learn) (1.23.5)
Requirement already satisfied: joblib>=1.1.1 in c:\users\wsaye\pycharmprojects\wmsayer.github.io\venv\lib\site-packages (from scikit-learn) (1.2.0)
Requirement already satisfied: scipy>=1.3.2 in c:\users\wsaye\pycharmprojects\wmsayer.github.io\venv\lib\site-packages (from scikit-learn) (1.9.3)
Requirement already satisfied: plotly in c:\users\wsaye\pycharmprojects\wmsayer.github.io\venv\lib\site-packages (5.11.0)
Requirement already satisfied: tenacity>=6.2.0 in c:\users\wsaye\pycharmprojects\wmsayer.github.io\venv\lib\site-packages (from plotly) (8.1.0)
import pandas as pd
import numpy as np
import json
import requests
import datetime as dt
from dateutil.relativedelta import relativedelta
import scipy
from sklearn.linear_model import LinearRegression
from sklearn.preprocessing import OneHotEncoder
from sklearn.model_selection import train_test_split
import sklearn.metrics as metrics
import matplotlib.pyplot as plt
import plotly.graph_objects as go
import plotly.express as px
from plotly.subplots import make_subplots
# import plotly.offline as pyo
# Set notebook mode to work in offline
# pyo.init_notebook_mode()
Data & File I/O#
JSON Handling#
Read JSON File#
def json_load(f_path):
"""Loads a local JSON file as a Python dict"""
with open(f_path) as f:
json_data = json.load(f)
return json_data
Flattening (JSON Normalize)#
json_norm_dict = [
{
"id": 1,
"name": "Cole Volk",
"fitness": {"height": 130, "weight": 60},
},
{"name": "Mark Reg", "fitness": {"height": 130, "weight": 60}},
{
"id": 2,
"name": "Faye Raker",
"fitness": {"height": 130, "weight": 60},
},
]
pd.json_normalize(json_norm_dict, max_level=0)
id | name | fitness | |
---|---|---|---|
0 | 1.0 | Cole Volk | {'height': 130, 'weight': 60} |
1 | NaN | Mark Reg | {'height': 130, 'weight': 60} |
2 | 2.0 | Faye Raker | {'height': 130, 'weight': 60} |
pd.json_normalize(json_norm_dict, max_level=1)
id | name | fitness.height | fitness.weight | |
---|---|---|---|---|
0 | 1.0 | Cole Volk | 130 | 60 |
1 | NaN | Mark Reg | 130 | 60 |
2 | 2.0 | Faye Raker | 130 | 60 |
json_norm_dict = [
{
"state": "Florida",
"shortname": "FL",
"info": {"governor": "Rick Scott"},
"counties": [
{"name": "Dade", "population": 12345},
{"name": "Broward", "population": 40000},
{"name": "Palm Beach", "population": 60000},
],
},
{
"state": "Ohio",
"shortname": "OH",
"info": {"governor": "John Kasich"},
"counties": [
{"name": "Summit", "population": 1234},
{"name": "Cuyahoga", "population": 1337},
],
},
]
pd.json_normalize(json_norm_dict,
record_path="counties",
meta=["state", "shortname", ["info", "governor"]])
name | population | state | shortname | info.governor | |
---|---|---|---|---|---|
0 | Dade | 12345 | Florida | FL | Rick Scott |
1 | Broward | 40000 | Florida | FL | Rick Scott |
2 | Palm Beach | 60000 | Florida | FL | Rick Scott |
3 | Summit | 1234 | Ohio | OH | John Kasich |
4 | Cuyahoga | 1337 | Ohio | OH | John Kasich |
Load JSON via REST API#
# make GET request to REST API and return as Python dict
def run_rest_get(url, params={}, headers={}, print_summ=True):
if print_summ:
print(line_break)
print("GET Address: %s\nHeaders %s:\nParameters %s:" % (url, repr(headers), repr(params)))
response = requests.get(url, params=params, headers=headers)
status_code = response.status_code
if status_code == 200:
json_dict = response.json()
else:
json_dict = {}
if print_summ:
print("Status Code: %d" % response.status_code)
if type(json_dict) == dict:
print("Response Keys: %s\n" % json_dict.keys())
return json_dict, status_code
Read SQL Query w/ Params#
def read_sql(f_path, params={}):
"""Reads a local text file (.sql) and inserts parameters if passed."""
with open(f_path) as f:
query = f.read()
if params:
query = query.format(**params)
return query
I/O tests#
# read JSON
# https://github.com/wmsayer/wmsayer.github.io/blob/master/docs/_bookfiles/data.json
test_json_path = "C:/Users/wsaye/PycharmProjects/wmsayer.github.io/docs/_bookfiles/data.json"
print(json_load(test_json_path))
{'api_key': 'fdghdfghfgfg'}
# read SQL (no params)
# https://github.com/wmsayer/wmsayer.github.io/blob/master/docs/_bookfiles/query.sql
test_sql_path = "C:/Users/wsaye/PycharmProjects/wmsayer.github.io/docs/_bookfiles/query.sql"
print(read_sql(test_sql_path))
SELECT *
FROM my_table
WHERE id = 1
# read SQL (w/ params)
# https://github.com/wmsayer/wmsayer.github.io/blob/master/docs/_bookfiles/query_w_params.sql
test_sql_params_path = "C:/Users/wsaye/PycharmProjects/wmsayer.github.io/docs/_bookfiles/query_w_params.sql"
test_params = {"my_id": 102393, "max_date": "2000/01/01"}
print(read_sql(test_sql_params_path, params=test_params))
SELECT *
FROM my_table
WHERE id = 102393 OR date = '2000/01/01'
Sample REST Dataset (CoinMetrics)#
def get_asset_metrics(assets, metrics, freq, page_size=10000, print_summ=True):
# freq options 1b, 1s, 1m, 1h, 1d
# for 'start_time' and 'end_time', formats "2006-01-20T00:00:00Z" and "2006-01-20" are supported among others
# https://docs.coinmetrics.io/api/v4#operation/getTimeseriesAssetMetrics
# https://docs.coinmetrics.io/info/metrics
assets_str = ", ".join(assets)
metrics_str = ", ".join(metrics)
api_root = 'https://community-api.coinmetrics.io/v4'
data_key = "data"
url = "/".join([api_root, "timeseries/asset-metrics"])
params = {'assets': assets_str, 'metrics': metrics_str, 'frequency': freq, 'page_size': page_size}
result_dict, status_code = run_rest_get(url, params=params, headers={}, print_summ=print_summ)
result_df = pd.DataFrame(result_dict[data_key])
result_df.sort_values(by=["asset", "time"], inplace=True)
result_df.reset_index(inplace=True, drop=True)
for m in metrics:
result_df[m] = result_df[m].astype(float)
return result_df
# basic method for pulling data from an API and storing in a local cache
# such that when you're debugging you only have to call the API once
def load_asset_metric_data(cache_path, pull_new=True):
if pull_new:
df = get_asset_metrics(test_assets, test_metrics, test_freq, print_summ=False)
df.to_csv(cache_path, index=False)
else:
df = pd.read_csv(cache_path)
return df
Get data w/ cache#
test_assets = ['btc', 'eth']
test_metrics = ['AdrActCnt', 'PriceUSD']
test_freq = '1d'
test_df_cache = "C:/Users/wsaye/PycharmProjects/CashAppInterview/data/cm_test_data.csv"
test_df = load_asset_metric_data(test_df_cache, pull_new=True)
test_df = test_df.dropna(subset=test_metrics).reset_index(drop=True)
test_df["datetime"] = pd.to_datetime(test_df["time"], utc=True) # str timestamp to datetime
test_df["dayname"] = test_df["datetime"].dt.day_name()
test_df["date"] = pd.to_datetime(test_df["time"], utc=True).dt.date # datetime to date
test_df
asset | time | AdrActCnt | PriceUSD | datetime | dayname | date | |
---|---|---|---|---|---|---|---|
0 | btc | 2010-07-18T00:00:00.000000000Z | 860.0 | 0.085840 | 2010-07-18 00:00:00+00:00 | Sunday | 2010-07-18 |
1 | btc | 2010-07-19T00:00:00.000000000Z | 929.0 | 0.080800 | 2010-07-19 00:00:00+00:00 | Monday | 2010-07-19 |
2 | btc | 2010-07-20T00:00:00.000000000Z | 936.0 | 0.074736 | 2010-07-20 00:00:00+00:00 | Tuesday | 2010-07-20 |
3 | btc | 2010-07-21T00:00:00.000000000Z | 784.0 | 0.079193 | 2010-07-21 00:00:00+00:00 | Wednesday | 2010-07-21 |
4 | btc | 2010-07-22T00:00:00.000000000Z | 594.0 | 0.058470 | 2010-07-22 00:00:00+00:00 | Thursday | 2010-07-22 |
... | ... | ... | ... | ... | ... | ... | ... |
7324 | eth | 2023-02-03T00:00:00.000000000Z | 457502.0 | 1665.605250 | 2023-02-03 00:00:00+00:00 | Friday | 2023-02-03 |
7325 | eth | 2023-02-04T00:00:00.000000000Z | 500596.0 | 1671.046952 | 2023-02-04 00:00:00+00:00 | Saturday | 2023-02-04 |
7326 | eth | 2023-02-05T00:00:00.000000000Z | 537666.0 | 1631.121142 | 2023-02-05 00:00:00+00:00 | Sunday | 2023-02-05 |
7327 | eth | 2023-02-06T00:00:00.000000000Z | 503556.0 | 1614.274822 | 2023-02-06 00:00:00+00:00 | Monday | 2023-02-06 |
7328 | eth | 2023-02-07T00:00:00.000000000Z | 477572.0 | 1672.722311 | 2023-02-07 00:00:00+00:00 | Tuesday | 2023-02-07 |
7329 rows × 7 columns
Pandas Options & Settings#
Docs and available options here
pd.get_option("display.max_rows")
pd.set_option("display.max_rows", 10)
pd.set_option("display.max_columns", 10)
Summarize Dataframe#
General Info#
test_df.describe() # summary stats of columns
AdrActCnt | PriceUSD | |
---|---|---|
count | 7.329000e+03 | 7329.000000 |
mean | 4.345369e+05 | 5753.152983 |
std | 3.559563e+05 | 12098.872294 |
min | 4.080000e+02 | 0.050541 |
25% | 1.013370e+05 | 121.930348 |
50% | 4.190120e+05 | 496.317744 |
75% | 6.759150e+05 | 4595.020018 |
max | 7.157228e+06 | 67541.755508 |
test_df.info() # dataframe schema info, column types
<class 'pandas.core.frame.DataFrame'>
RangeIndex: 7329 entries, 0 to 7328
Data columns (total 7 columns):
# Column Non-Null Count Dtype
--- ------ -------------- -----
0 asset 7329 non-null object
1 time 7329 non-null object
2 AdrActCnt 7329 non-null float64
3 PriceUSD 7329 non-null float64
4 datetime 7329 non-null datetime64[ns, UTC]
5 dayname 7329 non-null object
6 date 7329 non-null object
dtypes: datetime64[ns, UTC](1), float64(2), object(4)
memory usage: 400.9+ KB
test_df.dtypes
asset object
time object
AdrActCnt float64
PriceUSD float64
datetime datetime64[ns, UTC]
dayname object
date object
dtype: object
test_df.head()
asset | time | AdrActCnt | PriceUSD | datetime | dayname | date | |
---|---|---|---|---|---|---|---|
0 | btc | 2010-07-18T00:00:00.000000000Z | 860.0 | 0.085840 | 2010-07-18 00:00:00+00:00 | Sunday | 2010-07-18 |
1 | btc | 2010-07-19T00:00:00.000000000Z | 929.0 | 0.080800 | 2010-07-19 00:00:00+00:00 | Monday | 2010-07-19 |
2 | btc | 2010-07-20T00:00:00.000000000Z | 936.0 | 0.074736 | 2010-07-20 00:00:00+00:00 | Tuesday | 2010-07-20 |
3 | btc | 2010-07-21T00:00:00.000000000Z | 784.0 | 0.079193 | 2010-07-21 00:00:00+00:00 | Wednesday | 2010-07-21 |
4 | btc | 2010-07-22T00:00:00.000000000Z | 594.0 | 0.058470 | 2010-07-22 00:00:00+00:00 | Thursday | 2010-07-22 |
print(type(test_df.loc[0, "time"])) # type of particular entry
<class 'str'>
test_df.nlargest(5, "PriceUSD")
# test_df.nsmallest(5, "PriceUSD")
asset | time | AdrActCnt | PriceUSD | datetime | dayname | date | |
---|---|---|---|---|---|---|---|
4131 | btc | 2021-11-08T00:00:00.000000000Z | 1018796.0 | 67541.755508 | 2021-11-08 00:00:00+00:00 | Monday | 2021-11-08 |
4132 | btc | 2021-11-09T00:00:00.000000000Z | 1195638.0 | 67095.585671 | 2021-11-09 00:00:00+00:00 | Tuesday | 2021-11-09 |
4112 | btc | 2021-10-20T00:00:00.000000000Z | 977215.0 | 66061.796564 | 2021-10-20 00:00:00+00:00 | Wednesday | 2021-10-20 |
4137 | btc | 2021-11-14T00:00:00.000000000Z | 806451.0 | 65032.225655 | 2021-11-14 00:00:00+00:00 | Sunday | 2021-11-14 |
4134 | btc | 2021-11-11T00:00:00.000000000Z | 1037951.0 | 64962.931294 | 2021-11-11 00:00:00+00:00 | Thursday | 2021-11-11 |
test_df["asset"].unique()
# test_df["asset"].nunique()
array(['btc', 'eth'], dtype=object)
Crosstab#
cross_df = test_df.loc[test_df["asset"]== "btc", ["datetime", "dayname", "PriceUSD"]].copy().dropna()
cross_df = cross_df.sort_values(by="datetime")
cross_df["7d_SMA"] = cross_df["PriceUSD"].rolling(7).mean()
cross_df["beating_SMA"] = cross_df["PriceUSD"] > cross_df["7d_SMA"]
cross_df["return"] = cross_df["PriceUSD"].pct_change()
cross_df.dropna(inplace=True)
cross_df
datetime | dayname | PriceUSD | 7d_SMA | beating_SMA | return | |
---|---|---|---|---|---|---|
6 | 2010-07-24 00:00:00+00:00 | Saturday | 0.054540 | 0.070596 | False | -0.099894 |
7 | 2010-07-25 00:00:00+00:00 | Sunday | 0.050541 | 0.065553 | False | -0.073329 |
8 | 2010-07-26 00:00:00+00:00 | Monday | 0.056000 | 0.062010 | False | 0.108020 |
9 | 2010-07-27 00:00:00+00:00 | Tuesday | 0.058622 | 0.059708 | False | 0.046822 |
10 | 2010-07-28 00:00:00+00:00 | Wednesday | 0.058911 | 0.056811 | True | 0.004931 |
... | ... | ... | ... | ... | ... | ... |
4583 | 2023-02-03 00:00:00+00:00 | Friday | 23445.813177 | 23341.700805 | True | -0.002544 |
4584 | 2023-02-04 00:00:00+00:00 | Saturday | 23355.524386 | 23391.231508 | False | -0.003851 |
4585 | 2023-02-05 00:00:00+00:00 | Sunday | 22957.225191 | 23274.407054 | False | -0.017054 |
4586 | 2023-02-06 00:00:00+00:00 | Monday | 22745.165037 | 23266.655308 | False | -0.009237 |
4587 | 2023-02-07 00:00:00+00:00 | Tuesday | 23266.269204 | 23286.114921 | False | 0.022911 |
4582 rows × 6 columns
pd.crosstab(cross_df['beating_SMA'], cross_df['dayname'])
dayname | Friday | Monday | Saturday | Sunday | Thursday | Tuesday | Wednesday |
---|---|---|---|---|---|---|---|
beating_SMA | |||||||
False | 271 | 288 | 295 | 296 | 278 | 300 | 284 |
True | 383 | 367 | 360 | 359 | 376 | 355 | 370 |
pd.crosstab(cross_df['beating_SMA'], cross_df['dayname'], normalize=True)
dayname | Friday | Monday | Saturday | Sunday | Thursday | Tuesday | Wednesday |
---|---|---|---|---|---|---|---|
beating_SMA | |||||||
False | 0.059144 | 0.062855 | 0.064382 | 0.064601 | 0.060672 | 0.065474 | 0.061982 |
True | 0.083588 | 0.080096 | 0.078568 | 0.078350 | 0.082060 | 0.077477 | 0.080751 |
pd.crosstab(cross_df['beating_SMA'], cross_df['dayname'], values=cross_df['return'], aggfunc=np.mean)
dayname | Friday | Monday | Saturday | Sunday | Thursday | Tuesday | Wednesday |
---|---|---|---|---|---|---|---|
beating_SMA | |||||||
False | -0.018078 | -0.020847 | -0.012298 | -0.009072 | -0.020434 | -0.017126 | -0.017303 |
True | 0.019876 | 0.025667 | 0.017170 | 0.008909 | 0.021925 | 0.024923 | 0.022291 |
Sort/ Rank#
sort_df = test_df[["date", "asset", "PriceUSD"]].copy()
sort_df['price_rank'] = sort_df["PriceUSD"].rank(ascending=True, pct=False)
sort_df['price_pct'] = sort_df["PriceUSD"].rank(ascending=True, pct=True)
sort_df
date | asset | PriceUSD | price_rank | price_pct | |
---|---|---|---|---|---|
0 | 2010-07-18 | btc | 0.085840 | 83.0 | 0.011325 |
1 | 2010-07-19 | btc | 0.080800 | 81.0 | 0.011052 |
2 | 2010-07-20 | btc | 0.074736 | 79.0 | 0.010779 |
3 | 2010-07-21 | btc | 0.079193 | 80.0 | 0.010916 |
4 | 2010-07-22 | btc | 0.058470 | 5.0 | 0.000682 |
... | ... | ... | ... | ... | ... |
7324 | 2023-02-03 | eth | 1665.605250 | 4727.0 | 0.644972 |
7325 | 2023-02-04 | eth | 1671.046952 | 4728.0 | 0.645108 |
7326 | 2023-02-05 | eth | 1631.121142 | 4710.0 | 0.642652 |
7327 | 2023-02-06 | eth | 1614.274822 | 4699.0 | 0.641152 |
7328 | 2023-02-07 | eth | 1672.722311 | 4730.0 | 0.645381 |
7329 rows × 5 columns
sort_df.sort_values(by="price_rank", ascending=False)
date | asset | PriceUSD | price_rank | price_pct | |
---|---|---|---|---|---|
4131 | 2021-11-08 | btc | 67541.755508 | 7329.0 | 1.000000 |
4132 | 2021-11-09 | btc | 67095.585671 | 7328.0 | 0.999864 |
4112 | 2021-10-20 | btc | 66061.796564 | 7327.0 | 0.999727 |
4137 | 2021-11-14 | btc | 65032.225655 | 7326.0 | 0.999591 |
4134 | 2021-11-11 | btc | 64962.931294 | 7325.0 | 0.999454 |
... | ... | ... | ... | ... | ... |
4 | 2010-07-22 | btc | 0.058470 | 5.0 | 0.000682 |
17 | 2010-08-04 | btc | 0.057016 | 4.0 | 0.000546 |
8 | 2010-07-26 | btc | 0.056000 | 3.0 | 0.000409 |
6 | 2010-07-24 | btc | 0.054540 | 2.0 | 0.000273 |
7 | 2010-07-25 | btc | 0.050541 | 1.0 | 0.000136 |
7329 rows × 5 columns
Cleaning#
Deleting Rows/Columns here
Replace#
replace_df = test_df[["date", "asset", "dayname"]].copy()
# replace_df.replace("Sunday", "Sun")
replace_df.replace({"Sunday": "S", "Monday": "M", "Tuesday": "T"})
date | asset | dayname | |
---|---|---|---|
0 | 2010-07-18 | btc | S |
1 | 2010-07-19 | btc | M |
2 | 2010-07-20 | btc | T |
3 | 2010-07-21 | btc | Wednesday |
4 | 2010-07-22 | btc | Thursday |
... | ... | ... | ... |
7324 | 2023-02-03 | eth | Friday |
7325 | 2023-02-04 | eth | Saturday |
7326 | 2023-02-05 | eth | S |
7327 | 2023-02-06 | eth | M |
7328 | 2023-02-07 | eth | T |
7329 rows × 3 columns
Drop/Fill NA()#
cleaning_df = test_df[["date", "asset", "PriceUSD"]].pivot(index="date", columns="asset", values="PriceUSD")
cleaning_df
asset | btc | eth |
---|---|---|
date | ||
2010-07-18 | 0.085840 | NaN |
2010-07-19 | 0.080800 | NaN |
2010-07-20 | 0.074736 | NaN |
2010-07-21 | 0.079193 | NaN |
2010-07-22 | 0.058470 | NaN |
... | ... | ... |
2023-02-03 | 23445.813177 | 1665.605250 |
2023-02-04 | 23355.524386 | 1671.046952 |
2023-02-05 | 22957.225191 | 1631.121142 |
2023-02-06 | 22745.165037 | 1614.274822 |
2023-02-07 | 23266.269204 | 1672.722311 |
4588 rows × 2 columns
# cleaning_df.dropna() # drops N/A looking in all columns
cleaning_df.dropna(subset=["eth"]) # drops N/A in subset only
asset | btc | eth |
---|---|---|
date | ||
2015-08-08 | 261.450276 | 1.199990 |
2015-08-09 | 266.342020 | 1.199990 |
2015-08-10 | 264.928825 | 1.199990 |
2015-08-11 | 271.421736 | 0.990000 |
2015-08-12 | 268.143868 | 1.288000 |
... | ... | ... |
2023-02-03 | 23445.813177 | 1665.605250 |
2023-02-04 | 23355.524386 | 1671.046952 |
2023-02-05 | 22957.225191 | 1631.121142 |
2023-02-06 | 22745.165037 | 1614.274822 |
2023-02-07 | 23266.269204 | 1672.722311 |
2741 rows × 2 columns
cleaning_df.fillna(-1)
asset | btc | eth |
---|---|---|
date | ||
2010-07-18 | 0.085840 | -1.000000 |
2010-07-19 | 0.080800 | -1.000000 |
2010-07-20 | 0.074736 | -1.000000 |
2010-07-21 | 0.079193 | -1.000000 |
2010-07-22 | 0.058470 | -1.000000 |
... | ... | ... |
2023-02-03 | 23445.813177 | 1665.605250 |
2023-02-04 | 23355.524386 | 1671.046952 |
2023-02-05 | 22957.225191 | 1631.121142 |
2023-02-06 | 22745.165037 | 1614.274822 |
2023-02-07 | 23266.269204 | 1672.722311 |
4588 rows × 2 columns
cleaning_df.fillna(method="ffill")
asset | btc | eth |
---|---|---|
date | ||
2010-07-18 | 0.085840 | NaN |
2010-07-19 | 0.080800 | NaN |
2010-07-20 | 0.074736 | NaN |
2010-07-21 | 0.079193 | NaN |
2010-07-22 | 0.058470 | NaN |
... | ... | ... |
2023-02-03 | 23445.813177 | 1665.605250 |
2023-02-04 | 23355.524386 | 1671.046952 |
2023-02-05 | 22957.225191 | 1631.121142 |
2023-02-06 | 22745.165037 | 1614.274822 |
2023-02-07 | 23266.269204 | 1672.722311 |
4588 rows × 2 columns
cleaning_df.fillna(method="bfill")
asset | btc | eth |
---|---|---|
date | ||
2010-07-18 | 0.085840 | 1.199990 |
2010-07-19 | 0.080800 | 1.199990 |
2010-07-20 | 0.074736 | 1.199990 |
2010-07-21 | 0.079193 | 1.199990 |
2010-07-22 | 0.058470 | 1.199990 |
... | ... | ... |
2023-02-03 | 23445.813177 | 1665.605250 |
2023-02-04 | 23355.524386 | 1671.046952 |
2023-02-05 | 22957.225191 | 1631.121142 |
2023-02-06 | 22745.165037 | 1614.274822 |
2023-02-07 | 23266.269204 | 1672.722311 |
4588 rows × 2 columns
# setup df for interpolation
interp_df = cleaning_df.iloc[cleaning_df.shape[0] - 5:, :].copy()
interp_df["btc_og"] = interp_df["btc"]
interp_df["eth_og"] = interp_df["eth"]
interp_df.iloc[1, 0:2] = [np.nan ,np.nan]
interp_df.interpolate(method="linear")
asset | btc | eth | btc_og | eth_og |
---|---|---|---|---|
date | ||||
2023-02-03 | 23445.813177 | 1665.605250 | 23445.813177 | 1665.605250 |
2023-02-04 | 23201.519184 | 1648.363196 | 23355.524386 | 1671.046952 |
2023-02-05 | 22957.225191 | 1631.121142 | 22957.225191 | 1631.121142 |
2023-02-06 | 22745.165037 | 1614.274822 | 22745.165037 | 1614.274822 |
2023-02-07 | 23266.269204 | 1672.722311 | 23266.269204 | 1672.722311 |
Selecting/Sampling#
test_df.select_dtypes(include='float64')
AdrActCnt | PriceUSD | |
---|---|---|
0 | 860.0 | 0.085840 |
1 | 929.0 | 0.080800 |
2 | 936.0 | 0.074736 |
3 | 784.0 | 0.079193 |
4 | 594.0 | 0.058470 |
... | ... | ... |
7324 | 457502.0 | 1665.605250 |
7325 | 500596.0 | 1671.046952 |
7326 | 537666.0 | 1631.121142 |
7327 | 503556.0 | 1614.274822 |
7328 | 477572.0 | 1672.722311 |
7329 rows × 2 columns
# test_df.sample(n = 200)
test_df.sample(frac = 0.25, random_state=42)
asset | time | AdrActCnt | PriceUSD | datetime | dayname | date | |
---|---|---|---|---|---|---|---|
2576 | btc | 2017-08-06T00:00:00.000000000Z | 547079.0 | 3230.116709 | 2017-08-06 00:00:00+00:00 | Sunday | 2017-08-06 |
1565 | btc | 2014-10-30T00:00:00.000000000Z | 205736.0 | 344.609382 | 2014-10-30 00:00:00+00:00 | Thursday | 2014-10-30 |
865 | btc | 2012-11-29T00:00:00.000000000Z | 41205.0 | 12.497662 | 2012-11-29 00:00:00+00:00 | Thursday | 2012-11-29 |
4461 | btc | 2022-10-04T00:00:00.000000000Z | 932869.0 | 20339.971281 | 2022-10-04 00:00:00+00:00 | Tuesday | 2022-10-04 |
3728 | btc | 2020-10-01T00:00:00.000000000Z | 922880.0 | 10606.617333 | 2020-10-01 00:00:00+00:00 | Thursday | 2020-10-01 |
... | ... | ... | ... | ... | ... | ... | ... |
367 | btc | 2011-07-20T00:00:00.000000000Z | 25461.0 | 13.678331 | 2011-07-20 00:00:00+00:00 | Wednesday | 2011-07-20 |
3520 | btc | 2020-03-07T00:00:00.000000000Z | 682432.0 | 8898.306957 | 2020-03-07 00:00:00+00:00 | Saturday | 2020-03-07 |
5824 | eth | 2018-12-26T00:00:00.000000000Z | 288376.0 | 130.309451 | 2018-12-26 00:00:00+00:00 | Wednesday | 2018-12-26 |
4362 | btc | 2022-06-27T00:00:00.000000000Z | 953524.0 | 20751.051696 | 2022-06-27 00:00:00+00:00 | Monday | 2022-06-27 |
4280 | btc | 2022-04-06T00:00:00.000000000Z | 919776.0 | 43304.937199 | 2022-04-06 00:00:00+00:00 | Wednesday | 2022-04-06 |
1832 rows × 7 columns
Boolean Selection#
bool_df = test_df[["date", "asset", "PriceUSD"]].pivot(index="date", columns="asset", values="PriceUSD")
bool_df
asset | btc | eth |
---|---|---|
date | ||
2010-07-18 | 0.085840 | NaN |
2010-07-19 | 0.080800 | NaN |
2010-07-20 | 0.074736 | NaN |
2010-07-21 | 0.079193 | NaN |
2010-07-22 | 0.058470 | NaN |
... | ... | ... |
2023-02-03 | 23445.813177 | 1665.605250 |
2023-02-04 | 23355.524386 | 1671.046952 |
2023-02-05 | 22957.225191 | 1631.121142 |
2023-02-06 | 22745.165037 | 1614.274822 |
2023-02-07 | 23266.269204 | 1672.722311 |
4588 rows × 2 columns
# returns Series of same shape w/ np.NaN at failing rows (default)
# bool_df['PriceUSD'].where(bool_df['PriceUSD'] > 10**4) # returns np.Nan in failing rows
bool_df['eth'].where(bool_df['eth'] > 10**3, 0) # returns 0 in failing rows
date
2010-07-18 0.000000
2010-07-19 0.000000
2010-07-20 0.000000
2010-07-21 0.000000
2010-07-22 0.000000
...
2023-02-03 1665.605250
2023-02-04 1671.046952
2023-02-05 1631.121142
2023-02-06 1614.274822
2023-02-07 1672.722311
Name: eth, Length: 4588, dtype: float64
test_df["asset"].isin(["btc"])
0 True
1 True
2 True
3 True
4 True
...
7324 False
7325 False
7326 False
7327 False
7328 False
Name: asset, Length: 7329, dtype: bool
na_check_df = bool_df.isna()
na_check_series = na_check_df.any(axis=1) # aggregate booleans
bool_df.loc[na_check_series, :]
asset | btc | eth |
---|---|---|
date | ||
2010-07-18 | 0.085840 | NaN |
2010-07-19 | 0.080800 | NaN |
2010-07-20 | 0.074736 | NaN |
2010-07-21 | 0.079193 | NaN |
2010-07-22 | 0.058470 | NaN |
... | ... | ... |
2015-08-03 | 282.185052 | NaN |
2015-08-04 | 285.286617 | NaN |
2015-08-05 | 282.338887 | NaN |
2015-08-06 | 278.995749 | NaN |
2015-08-07 | 279.488715 | NaN |
1847 rows × 2 columns
bool_df.loc[(bool_df['eth'] > 10**3), :]
asset | btc | eth |
---|---|---|
date | ||
2018-01-06 | 17103.589280 | 1006.157475 |
2018-01-07 | 16231.694999 | 1102.889537 |
2018-01-08 | 14937.415089 | 1134.699060 |
2018-01-09 | 14378.586217 | 1288.406875 |
2018-01-10 | 14669.088266 | 1231.767295 |
... | ... | ... |
2023-02-03 | 23445.813177 | 1665.605250 |
2023-02-04 | 23355.524386 | 1671.046952 |
2023-02-05 | 22957.225191 | 1631.121142 |
2023-02-06 | 22745.165037 | 1614.274822 |
2023-02-07 | 23266.269204 | 1672.722311 |
789 rows × 2 columns
Boolean Operators#
bool_df.loc[~(bool_df['eth'] > 10**3), :] # NOT
asset | btc | eth |
---|---|---|
date | ||
2010-07-18 | 0.085840 | NaN |
2010-07-19 | 0.080800 | NaN |
2010-07-20 | 0.074736 | NaN |
2010-07-21 | 0.079193 | NaN |
2010-07-22 | 0.058470 | NaN |
... | ... | ... |
2020-12-31 | 29022.671413 | 739.025850 |
2021-01-01 | 29380.693733 | 730.914321 |
2021-01-02 | 32022.681058 | 775.296622 |
2021-01-03 | 33277.835305 | 990.365325 |
2022-06-18 | 19013.867254 | 992.790097 |
3799 rows × 2 columns
bool_df.loc[(bool_df['eth'] > 10**3) & (bool_df['eth'] > 10), :] # AND
asset | btc | eth |
---|---|---|
date | ||
2018-01-06 | 17103.589280 | 1006.157475 |
2018-01-07 | 16231.694999 | 1102.889537 |
2018-01-08 | 14937.415089 | 1134.699060 |
2018-01-09 | 14378.586217 | 1288.406875 |
2018-01-10 | 14669.088266 | 1231.767295 |
... | ... | ... |
2023-02-03 | 23445.813177 | 1665.605250 |
2023-02-04 | 23355.524386 | 1671.046952 |
2023-02-05 | 22957.225191 | 1631.121142 |
2023-02-06 | 22745.165037 | 1614.274822 |
2023-02-07 | 23266.269204 | 1672.722311 |
789 rows × 2 columns
bool_df.loc[(bool_df['eth'] > 10**3) | (bool_df['eth'] > 10), :] # OR
asset | btc | eth |
---|---|---|
date | ||
2016-03-05 | 397.314454 | 10.751006 |
2016-03-06 | 403.705187 | 10.984407 |
2016-03-09 | 412.540704 | 11.817099 |
2016-03-10 | 416.340929 | 11.165651 |
2016-03-11 | 419.511934 | 11.122905 |
... | ... | ... |
2023-02-03 | 23445.813177 | 1665.605250 |
2023-02-04 | 23355.524386 | 1671.046952 |
2023-02-05 | 22957.225191 | 1631.121142 |
2023-02-06 | 22745.165037 | 1614.274822 |
2023-02-07 | 23266.269204 | 1672.722311 |
2438 rows × 2 columns
Datetime#
Python datetime <-> string formatting here
Datetime to string#
Python:
test_date_str = "2022-01-01"
print(dt.datetime.strptime(test_date_str, "%Y-%m-%d"))
print(dt.datetime.strptime(test_date_str, "%Y-%m-%d").date())
2022-01-01 00:00:00
2022-01-01
Pandas:
test_df["datetime"] = pd.to_datetime(test_df["time"], utc=True) # str timestamp to datetime
test_df['datetime_alt'] = pd.to_datetime(test_df["time"], format='%Y-%m-%dT%H:%M:%S.%fZ', utc=True)
test_df["date"] = pd.to_datetime(test_df["time"], utc=True).dt.date # datetime to date
test_df['datetime_str'] = test_df["datetime"].dt.strftime('%Y-%m-%dT%H:%M:%S.%fZ') # datetime to str
test_df['date_str'] = pd.to_datetime(test_df["date"]).dt.strftime('%Y-%m-%d') # date to str
test_df[["datetime", "datetime_alt", "date", "datetime_str", "date_str"]]
datetime | datetime_alt | date | datetime_str | date_str | |
---|---|---|---|---|---|
0 | 2010-07-18 00:00:00+00:00 | 2010-07-18 00:00:00+00:00 | 2010-07-18 | 2010-07-18T00:00:00.000000Z | 2010-07-18 |
1 | 2010-07-19 00:00:00+00:00 | 2010-07-19 00:00:00+00:00 | 2010-07-19 | 2010-07-19T00:00:00.000000Z | 2010-07-19 |
2 | 2010-07-20 00:00:00+00:00 | 2010-07-20 00:00:00+00:00 | 2010-07-20 | 2010-07-20T00:00:00.000000Z | 2010-07-20 |
3 | 2010-07-21 00:00:00+00:00 | 2010-07-21 00:00:00+00:00 | 2010-07-21 | 2010-07-21T00:00:00.000000Z | 2010-07-21 |
4 | 2010-07-22 00:00:00+00:00 | 2010-07-22 00:00:00+00:00 | 2010-07-22 | 2010-07-22T00:00:00.000000Z | 2010-07-22 |
... | ... | ... | ... | ... | ... |
7324 | 2023-02-03 00:00:00+00:00 | 2023-02-03 00:00:00+00:00 | 2023-02-03 | 2023-02-03T00:00:00.000000Z | 2023-02-03 |
7325 | 2023-02-04 00:00:00+00:00 | 2023-02-04 00:00:00+00:00 | 2023-02-04 | 2023-02-04T00:00:00.000000Z | 2023-02-04 |
7326 | 2023-02-05 00:00:00+00:00 | 2023-02-05 00:00:00+00:00 | 2023-02-05 | 2023-02-05T00:00:00.000000Z | 2023-02-05 |
7327 | 2023-02-06 00:00:00+00:00 | 2023-02-06 00:00:00+00:00 | 2023-02-06 | 2023-02-06T00:00:00.000000Z | 2023-02-06 |
7328 | 2023-02-07 00:00:00+00:00 | 2023-02-07 00:00:00+00:00 | 2023-02-07 | 2023-02-07T00:00:00.000000Z | 2023-02-07 |
7329 rows × 5 columns
Datetime Altering#
Python:
test_date_str = "2022-01-01"
test_dt = dt.datetime.strptime(test_date_str, "%Y-%m-%d")
print(test_dt + dt.timedelta(days=2))
print(test_dt + relativedelta(years=5, months=4))
2022-01-03 00:00:00
2027-05-01 00:00:00
Pandas:
# (also pd.Timedelta)
test_df["date_offset"] = test_df["date"] + pd.DateOffset(years=2, months=2, days=1)
test_df[["date", "date_offset"]]
date | date_offset | |
---|---|---|
0 | 2010-07-18 | 2012-09-19 |
1 | 2010-07-19 | 2012-09-20 |
2 | 2010-07-20 | 2012-09-21 |
3 | 2010-07-21 | 2012-09-22 |
4 | 2010-07-22 | 2012-09-23 |
... | ... | ... |
7324 | 2023-02-03 | 2025-04-04 |
7325 | 2023-02-04 | 2025-04-05 |
7326 | 2023-02-05 | 2025-04-06 |
7327 | 2023-02-06 | 2025-04-07 |
7328 | 2023-02-07 | 2025-04-08 |
7329 rows × 2 columns
Date Parts (Week & Weekday)#
Python:
test_date_str = "2022-01-01"
test_dt = dt.datetime.strptime(test_date_str, "%Y-%m-%d")
print(test_dt.isocalendar().week)
print(test_dt.weekday())
print(test_dt.strftime('%A')) # get day name
52
5
Saturday
Pandas:
test_df["week"] = test_df["datetime"].dt.isocalendar().week
test_df["weekday"] = test_df["datetime"].dt.weekday
test_df["dayname"] = test_df["datetime"].dt.day_name()
test_df["monthname"] = test_df["datetime"].dt.month_name()
test_df["year"] = test_df["datetime"].dt.year
test_df[["date", "week", "weekday", "dayname", "monthname", "year"]]
date | week | weekday | dayname | monthname | year | |
---|---|---|---|---|---|---|
0 | 2010-07-18 | 28 | 6 | Sunday | July | 2010 |
1 | 2010-07-19 | 29 | 0 | Monday | July | 2010 |
2 | 2010-07-20 | 29 | 1 | Tuesday | July | 2010 |
3 | 2010-07-21 | 29 | 2 | Wednesday | July | 2010 |
4 | 2010-07-22 | 29 | 3 | Thursday | July | 2010 |
... | ... | ... | ... | ... | ... | ... |
7324 | 2023-02-03 | 5 | 4 | Friday | February | 2023 |
7325 | 2023-02-04 | 5 | 5 | Saturday | February | 2023 |
7326 | 2023-02-05 | 5 | 6 | Sunday | February | 2023 |
7327 | 2023-02-06 | 6 | 0 | Monday | February | 2023 |
7328 | 2023-02-07 | 6 | 1 | Tuesday | February | 2023 |
7329 rows × 6 columns
Numerical#
num_df = test_df.loc[3000:, ["date", "asset", "PriceUSD"]].copy()
num_df["PriceUSD_rnd"] = num_df["PriceUSD"].round()
num_df["PriceUSD_rnd1"] = num_df["PriceUSD"].round(1)
num_df["PriceUSD_floor"] = np.floor(num_df["PriceUSD"])
num_df["PriceUSD_ceil"] = np.ceil(num_df["PriceUSD"])
num_df
date | asset | PriceUSD | PriceUSD_rnd | PriceUSD_rnd1 | PriceUSD_floor | PriceUSD_ceil | |
---|---|---|---|---|---|---|---|
3000 | 2018-10-04 | btc | 6545.285668 | 6545.0 | 6545.3 | 6545.0 | 6546.0 |
3001 | 2018-10-05 | btc | 6585.149580 | 6585.0 | 6585.1 | 6585.0 | 6586.0 |
3002 | 2018-10-06 | btc | 6550.478316 | 6550.0 | 6550.5 | 6550.0 | 6551.0 |
3003 | 2018-10-07 | btc | 6564.568260 | 6565.0 | 6564.6 | 6564.0 | 6565.0 |
3004 | 2018-10-08 | btc | 6604.685274 | 6605.0 | 6604.7 | 6604.0 | 6605.0 |
... | ... | ... | ... | ... | ... | ... | ... |
7324 | 2023-02-03 | eth | 1665.605250 | 1666.0 | 1665.6 | 1665.0 | 1666.0 |
7325 | 2023-02-04 | eth | 1671.046952 | 1671.0 | 1671.0 | 1671.0 | 1672.0 |
7326 | 2023-02-05 | eth | 1631.121142 | 1631.0 | 1631.1 | 1631.0 | 1632.0 |
7327 | 2023-02-06 | eth | 1614.274822 | 1614.0 | 1614.3 | 1614.0 | 1615.0 |
7328 | 2023-02-07 | eth | 1672.722311 | 1673.0 | 1672.7 | 1672.0 | 1673.0 |
4329 rows × 7 columns
Transforms#
Indexes#
df.set_index(keys, drop=True, verify_integrity=False)
df.reset_index(drop=False)
df.reindex()
Pivot & Melt#
pd.unstack() - pivot multilevel index
Pivot to MultiIndex#
pivot_df = test_df.pivot(index="date", columns="asset", values=['AdrActCnt', 'PriceUSD'])
pivot_df.reset_index(drop=False, inplace=True)
pivot_df
date | AdrActCnt | PriceUSD | |||
---|---|---|---|---|---|
asset | btc | eth | btc | eth | |
0 | 2010-07-18 | 860.0 | NaN | 0.085840 | NaN |
1 | 2010-07-19 | 929.0 | NaN | 0.080800 | NaN |
2 | 2010-07-20 | 936.0 | NaN | 0.074736 | NaN |
3 | 2010-07-21 | 784.0 | NaN | 0.079193 | NaN |
4 | 2010-07-22 | 594.0 | NaN | 0.058470 | NaN |
... | ... | ... | ... | ... | ... |
4583 | 2023-02-03 | 988867.0 | 457502.0 | 23445.813177 | 1665.605250 |
4584 | 2023-02-04 | 961115.0 | 500596.0 | 23355.524386 | 1671.046952 |
4585 | 2023-02-05 | 802171.0 | 537666.0 | 22957.225191 | 1631.121142 |
4586 | 2023-02-06 | 920525.0 | 503556.0 | 22745.165037 | 1614.274822 |
4587 | 2023-02-07 | 944049.0 | 477572.0 | 23266.269204 | 1672.722311 |
4588 rows × 5 columns
Melt from MultiIndex#
# pivot_df = pd.melt(pivot_df, col_level=0, id_vars=["date"])
pivot_df = pd.melt(pivot_df, id_vars=[("date", "")])
pivot_df.columns = ["date", "metric", "asset", "value"]
pivot_df
date | metric | asset | value | |
---|---|---|---|---|
0 | 2010-07-18 | AdrActCnt | btc | 860.000000 |
1 | 2010-07-19 | AdrActCnt | btc | 929.000000 |
2 | 2010-07-20 | AdrActCnt | btc | 936.000000 |
3 | 2010-07-21 | AdrActCnt | btc | 784.000000 |
4 | 2010-07-22 | AdrActCnt | btc | 594.000000 |
... | ... | ... | ... | ... |
18347 | 2023-02-03 | PriceUSD | eth | 1665.605250 |
18348 | 2023-02-04 | PriceUSD | eth | 1671.046952 |
18349 | 2023-02-05 | PriceUSD | eth | 1631.121142 |
18350 | 2023-02-06 | PriceUSD | eth | 1614.274822 |
18351 | 2023-02-07 | PriceUSD | eth | 1672.722311 |
18352 rows × 4 columns
Pivot back to OG (single Index)#
pivot_df = pivot_df.pivot(index=["date", "asset"], columns="metric", values="value")
pivot_df.columns = pivot_df.columns.rename("")
pivot_df.reset_index(drop=False, inplace=True)
pivot_df
date | asset | AdrActCnt | PriceUSD | |
---|---|---|---|---|
0 | 2010-07-18 | btc | 860.0 | 0.085840 |
1 | 2010-07-18 | eth | NaN | NaN |
2 | 2010-07-19 | btc | 929.0 | 0.080800 |
3 | 2010-07-19 | eth | NaN | NaN |
4 | 2010-07-20 | btc | 936.0 | 0.074736 |
... | ... | ... | ... | ... |
9171 | 2023-02-05 | eth | 537666.0 | 1631.121142 |
9172 | 2023-02-06 | btc | 920525.0 | 22745.165037 |
9173 | 2023-02-06 | eth | 503556.0 | 1614.274822 |
9174 | 2023-02-07 | btc | 944049.0 | 23266.269204 |
9175 | 2023-02-07 | eth | 477572.0 | 1672.722311 |
9176 rows × 4 columns
Pivot to Date Index (Fill missing dates)#
date_rng = pd.date_range(test_df["date"].min(), test_df["date"].max())
pivot_fill_df = test_df.pivot(index="date", columns="asset", values='PriceUSD')
# pivot_fill_df = pivot_fill_df.drop(labels=[1, 3, 4524], axis=0) # drop by index value
pivot_fill_df = pivot_fill_df.drop(pivot_fill_df.index[[1, 3, 4524]]) # drop by row num
pivot_fill_df = pivot_fill_df.reindex(date_rng, fill_value=np.nan)
pivot_fill_df
asset | btc | eth |
---|---|---|
2010-07-18 | 0.085840 | NaN |
2010-07-19 | NaN | NaN |
2010-07-20 | 0.074736 | NaN |
2010-07-21 | NaN | NaN |
2010-07-22 | 0.058470 | NaN |
... | ... | ... |
2023-02-03 | 23445.813177 | 1665.605250 |
2023-02-04 | 23355.524386 | 1671.046952 |
2023-02-05 | 22957.225191 | 1631.121142 |
2023-02-06 | 22745.165037 | 1614.274822 |
2023-02-07 | 23266.269204 | 1672.722311 |
4588 rows × 2 columns
Join & Merge#
article on when to use join vs merge
Merge:
can join on indices or columns
validate - check 1:1, 1:many, etc. (also available for join)
indicator - produces additional column to indicate “left_only”, “right_only”, or “both”
join_merge_df = test_df.loc[3000:, ["date", "datetime", "asset", 'AdrActCnt', 'PriceUSD']].copy()
join_merge_df = join_merge_df.sort_values(by="date").reset_index(drop=True)
join_merge_df
date | datetime | asset | AdrActCnt | PriceUSD | |
---|---|---|---|---|---|
0 | 2015-08-08 | 2015-08-08 00:00:00+00:00 | eth | 1208.0 | 1.199990 |
1 | 2015-08-09 | 2015-08-09 00:00:00+00:00 | eth | 1113.0 | 1.199990 |
2 | 2015-08-10 | 2015-08-10 00:00:00+00:00 | eth | 1430.0 | 1.199990 |
3 | 2015-08-11 | 2015-08-11 00:00:00+00:00 | eth | 2697.0 | 0.990000 |
4 | 2015-08-12 | 2015-08-12 00:00:00+00:00 | eth | 1219.0 | 1.288000 |
... | ... | ... | ... | ... | ... |
4324 | 2023-02-05 | 2023-02-05 00:00:00+00:00 | eth | 537666.0 | 1631.121142 |
4325 | 2023-02-06 | 2023-02-06 00:00:00+00:00 | eth | 503556.0 | 1614.274822 |
4326 | 2023-02-06 | 2023-02-06 00:00:00+00:00 | btc | 920525.0 | 22745.165037 |
4327 | 2023-02-07 | 2023-02-07 00:00:00+00:00 | btc | 944049.0 | 23266.269204 |
4328 | 2023-02-07 | 2023-02-07 00:00:00+00:00 | eth | 477572.0 | 1672.722311 |
4329 rows × 5 columns
join_cols = ["date", "asset"]
join_merge_df1 = join_merge_df.loc[:3000, join_cols + ["AdrActCnt"]]
join_merge_df2 = join_merge_df.loc[:, join_cols + ["PriceUSD"]]
print(join_merge_df1)
print(join_merge_df2)
date asset AdrActCnt
0 2015-08-08 eth 1208.0
1 2015-08-09 eth 1113.0
2 2015-08-10 eth 1430.0
3 2015-08-11 eth 2697.0
4 2015-08-12 eth 1219.0
... ... ... ...
2996 2021-04-12 eth 649920.0
2997 2021-04-13 btc 1178027.0
2998 2021-04-13 eth 641476.0
2999 2021-04-14 eth 662089.0
3000 2021-04-14 btc 1149773.0
[3001 rows x 3 columns]
date asset PriceUSD
0 2015-08-08 eth 1.199990
1 2015-08-09 eth 1.199990
2 2015-08-10 eth 1.199990
3 2015-08-11 eth 0.990000
4 2015-08-12 eth 1.288000
... ... ... ...
4324 2023-02-05 eth 1631.121142
4325 2023-02-06 eth 1614.274822
4326 2023-02-06 btc 22745.165037
4327 2023-02-07 btc 23266.269204
4328 2023-02-07 eth 1672.722311
[4329 rows x 3 columns]
joined_df = join_merge_df1.join(join_merge_df2.set_index(keys=join_cols), how="outer", on=join_cols)
joined_df
date | asset | AdrActCnt | PriceUSD | |
---|---|---|---|---|
0 | 2015-08-08 | eth | 1208.0 | 1.199990 |
1 | 2015-08-09 | eth | 1113.0 | 1.199990 |
2 | 2015-08-10 | eth | 1430.0 | 1.199990 |
3 | 2015-08-11 | eth | 2697.0 | 0.990000 |
4 | 2015-08-12 | eth | 1219.0 | 1.288000 |
... | ... | ... | ... | ... |
3000 | 2023-02-05 | eth | NaN | 1631.121142 |
3000 | 2023-02-06 | eth | NaN | 1614.274822 |
3000 | 2023-02-06 | btc | NaN | 22745.165037 |
3000 | 2023-02-07 | btc | NaN | 23266.269204 |
3000 | 2023-02-07 | eth | NaN | 1672.722311 |
4329 rows × 4 columns
merged_df = join_merge_df1.merge(join_merge_df2, how="outer", on=join_cols, indicator=True)
merged_df
date | asset | AdrActCnt | PriceUSD | _merge | |
---|---|---|---|---|---|
0 | 2015-08-08 | eth | 1208.0 | 1.199990 | both |
1 | 2015-08-09 | eth | 1113.0 | 1.199990 | both |
2 | 2015-08-10 | eth | 1430.0 | 1.199990 | both |
3 | 2015-08-11 | eth | 2697.0 | 0.990000 | both |
4 | 2015-08-12 | eth | 1219.0 | 1.288000 | both |
... | ... | ... | ... | ... | ... |
4324 | 2023-02-05 | eth | NaN | 1631.121142 | right_only |
4325 | 2023-02-06 | eth | NaN | 1614.274822 | right_only |
4326 | 2023-02-06 | btc | NaN | 22745.165037 | right_only |
4327 | 2023-02-07 | btc | NaN | 23266.269204 | right_only |
4328 | 2023-02-07 | eth | NaN | 1672.722311 | right_only |
4329 rows × 5 columns
Explode#
explode_df = pd.DataFrame({"city": ['A', 'B', 'C'],
"day1": [22, 25, 21],
'day2':[31, 12, 67],
'day3': [27, 20, 15],
'day4': [34, 37, [41, 45, 67, 90, 21]],
'day5': [23, 54, 36]})
explode_df
city | day1 | day2 | day3 | day4 | day5 | |
---|---|---|---|---|---|---|
0 | A | 22 | 31 | 27 | 34 | 23 |
1 | B | 25 | 12 | 20 | 37 | 54 |
2 | C | 21 | 67 | 15 | [41, 45, 67, 90, 21] | 36 |
explode_df.explode("day4", ignore_index=False)
city | day1 | day2 | day3 | day4 | day5 | |
---|---|---|---|---|---|---|
0 | A | 22 | 31 | 27 | 34 | 23 |
1 | B | 25 | 12 | 20 | 37 | 54 |
2 | C | 21 | 67 | 15 | 41 | 36 |
2 | C | 21 | 67 | 15 | 45 | 36 |
2 | C | 21 | 67 | 15 | 67 | 36 |
2 | C | 21 | 67 | 15 | 90 | 36 |
2 | C | 21 | 67 | 15 | 21 | 36 |
Aggregation#
Aggregation functions#
mean(): Compute mean of groups
sum(): Compute sum of group values
size(): Compute group sizes
count(): Compute count of group
std(): Standard deviation of groups
var(): Compute variance of groups
sem(): Standard error of the mean of groups
first(): Compute first of group values
last(): Compute last of group values
nth() : Take nth value, or a subset if n is a list
min(): Compute min of group values
max(): Compute max of group values
agg_df = test_df[test_metrics]
agg_df.count()
AdrActCnt 7329
PriceUSD 7329
dtype: int64
agg_df.nunique()
AdrActCnt 7268
PriceUSD 7278
dtype: int64
agg_df.median()
AdrActCnt 419012.000000
PriceUSD 496.317744
dtype: float64
agg_df.quantile(q=0.10)
AdrActCnt 14817.800000
PriceUSD 5.596396
Name: 0.1, dtype: float64
Groupby#
DataFrame.groupby(by=None, axis=0, level=None, as_index=True, sort=True, group_keys=_NoDefault.no_default, observed=False, dropna=True)
group_df = test_df[["date", "year", "asset"] + test_metrics]
group_df.groupby(by="asset").nunique()
date | year | AdrActCnt | PriceUSD | |
---|---|---|---|---|
asset | ||||
btc | 4588 | 14 | 4562 | 4565 |
eth | 2741 | 9 | 2725 | 2716 |
group_df.groupby(by=["year", "asset"]).nunique()
date | AdrActCnt | PriceUSD | ||
---|---|---|---|---|
year | asset | |||
2010 | btc | 167 | 151 | 154 |
2011 | btc | 365 | 363 | 356 |
2012 | btc | 366 | 363 | 366 |
2013 | btc | 365 | 365 | 365 |
2014 | btc | 365 | 365 | 365 |
... | ... | ... | ... | ... |
2021 | eth | 365 | 365 | 365 |
2022 | btc | 365 | 365 | 365 |
eth | 365 | 364 | 365 | |
2023 | btc | 38 | 38 | 38 |
eth | 38 | 38 | 38 |
23 rows × 3 columns
Groupby Map#
agg_map = {'AdrActCnt':['count', 'nunique'],
'PriceUSD':['max', 'min', lambda x: x.max() - x.min()]
}
group_df.groupby(by=["year", "asset"]).agg(agg_map)
AdrActCnt | PriceUSD | |||||
---|---|---|---|---|---|---|
count | nunique | max | min | <lambda_0> | ||
year | asset | |||||
2010 | btc | 167 | 151 | 0.400982 | 0.050541 | 0.350442 |
2011 | btc | 365 | 363 | 29.029921 | 0.295000 | 28.734921 |
2012 | btc | 366 | 363 | 13.755252 | 4.255239 | 9.500012 |
2013 | btc | 365 | 365 | 1134.932231 | 13.280607 | 1121.651624 |
2014 | btc | 365 | 365 | 914.459961 | 310.442004 | 604.017957 |
... | ... | ... | ... | ... | ... | ... |
2021 | eth | 365 | 365 | 4811.156463 | 730.914321 | 4080.242142 |
2022 | btc | 365 | 365 | 47560.009382 | 15758.291282 | 31801.718100 |
eth | 365 | 364 | 3832.365610 | 992.790097 | 2839.575513 | |
2023 | btc | 38 | 38 | 23774.996368 | 16606.752043 | 7168.244325 |
eth | 38 | 38 | 1672.722311 | 1200.005919 | 472.716392 |
23 rows × 5 columns
Groupby Datetime#
DataFrame.groupby(pd.Grouper(key=”dfgfgdf”, axis=0, freq=’M’))
group_dt_df = test_df.pivot(index="datetime", columns="asset", values=test_metrics)
group_dt_df
AdrActCnt | PriceUSD | |||
---|---|---|---|---|
asset | btc | eth | btc | eth |
datetime | ||||
2010-07-18 00:00:00+00:00 | 860.0 | NaN | 0.085840 | NaN |
2010-07-19 00:00:00+00:00 | 929.0 | NaN | 0.080800 | NaN |
2010-07-20 00:00:00+00:00 | 936.0 | NaN | 0.074736 | NaN |
2010-07-21 00:00:00+00:00 | 784.0 | NaN | 0.079193 | NaN |
2010-07-22 00:00:00+00:00 | 594.0 | NaN | 0.058470 | NaN |
... | ... | ... | ... | ... |
2023-02-03 00:00:00+00:00 | 988867.0 | 457502.0 | 23445.813177 | 1665.605250 |
2023-02-04 00:00:00+00:00 | 961115.0 | 500596.0 | 23355.524386 | 1671.046952 |
2023-02-05 00:00:00+00:00 | 802171.0 | 537666.0 | 22957.225191 | 1631.121142 |
2023-02-06 00:00:00+00:00 | 920525.0 | 503556.0 | 22745.165037 | 1614.274822 |
2023-02-07 00:00:00+00:00 | 944049.0 | 477572.0 | 23266.269204 | 1672.722311 |
4588 rows × 4 columns
group_dt_df.groupby(pd.Grouper(axis=0, freq='M')).last()
AdrActCnt | PriceUSD | |||
---|---|---|---|---|
asset | btc | eth | btc | eth |
datetime | ||||
2010-07-31 00:00:00+00:00 | 479.0 | NaN | 0.067546 | NaN |
2010-08-31 00:00:00+00:00 | 733.0 | NaN | 0.060000 | NaN |
2010-09-30 00:00:00+00:00 | 640.0 | NaN | 0.061900 | NaN |
2010-10-31 00:00:00+00:00 | 609.0 | NaN | 0.192500 | NaN |
2010-11-30 00:00:00+00:00 | 817.0 | NaN | 0.208200 | NaN |
... | ... | ... | ... | ... |
2022-10-31 00:00:00+00:00 | 980614.0 | 476137.0 | 20490.858181 | 1571.203871 |
2022-11-30 00:00:00+00:00 | 946754.0 | 525545.0 | 17176.898691 | 1298.039420 |
2022-12-31 00:00:00+00:00 | 839910.0 | 519101.0 | 16524.217802 | 1195.330879 |
2023-01-31 00:00:00+00:00 | 944348.0 | 519409.0 | 23130.051913 | 1584.821083 |
2023-02-28 00:00:00+00:00 | 944049.0 | 477572.0 | 23266.269204 | 1672.722311 |
152 rows × 4 columns
group_dt_df.groupby(pd.Grouper(axis=0, freq='Y')).first()
AdrActCnt | PriceUSD | |||
---|---|---|---|---|
asset | btc | eth | btc | eth |
datetime | ||||
2010-12-31 00:00:00+00:00 | 860.0 | NaN | 0.085840 | NaN |
2011-12-31 00:00:00+00:00 | 1071.0 | NaN | 0.300000 | NaN |
2012-12-31 00:00:00+00:00 | 11474.0 | NaN | 5.294843 | NaN |
2013-12-31 00:00:00+00:00 | 38733.0 | NaN | 13.331371 | NaN |
2014-12-31 00:00:00+00:00 | 96516.0 | NaN | 752.404550 | NaN |
... | ... | ... | ... | ... |
2019-12-31 00:00:00+00:00 | 433715.0 | 227755.0 | 3808.117832 | 139.154644 |
2020-12-31 00:00:00+00:00 | 524360.0 | 231794.0 | 7170.631869 | 129.963875 |
2021-12-31 00:00:00+00:00 | 1001890.0 | 511250.0 | 29380.693733 | 730.914321 |
2022-12-31 00:00:00+00:00 | 695722.0 | 581311.0 | 47560.009382 | 3761.059640 |
2023-12-31 00:00:00+00:00 | 719716.0 | 522461.0 | 16606.752043 | 1200.005919 |
14 rows × 4 columns
group_dt_df_2 = test_df[["datetime", "asset", "AdrActCnt"]]
group_dt_df_2
datetime | asset | AdrActCnt | |
---|---|---|---|
0 | 2010-07-18 00:00:00+00:00 | btc | 860.0 |
1 | 2010-07-19 00:00:00+00:00 | btc | 929.0 |
2 | 2010-07-20 00:00:00+00:00 | btc | 936.0 |
3 | 2010-07-21 00:00:00+00:00 | btc | 784.0 |
4 | 2010-07-22 00:00:00+00:00 | btc | 594.0 |
... | ... | ... | ... |
7324 | 2023-02-03 00:00:00+00:00 | eth | 457502.0 |
7325 | 2023-02-04 00:00:00+00:00 | eth | 500596.0 |
7326 | 2023-02-05 00:00:00+00:00 | eth | 537666.0 |
7327 | 2023-02-06 00:00:00+00:00 | eth | 503556.0 |
7328 | 2023-02-07 00:00:00+00:00 | eth | 477572.0 |
7329 rows × 3 columns
group_dt_df_2.groupby(["asset", pd.Grouper(key="datetime", freq='Y')]).first()
AdrActCnt | ||
---|---|---|
asset | datetime | |
btc | 2010-12-31 00:00:00+00:00 | 860.0 |
2011-12-31 00:00:00+00:00 | 1071.0 | |
2012-12-31 00:00:00+00:00 | 11474.0 | |
2013-12-31 00:00:00+00:00 | 38733.0 | |
2014-12-31 00:00:00+00:00 | 96516.0 | |
... | ... | ... |
eth | 2019-12-31 00:00:00+00:00 | 227755.0 |
2020-12-31 00:00:00+00:00 | 231794.0 | |
2021-12-31 00:00:00+00:00 | 511250.0 | |
2022-12-31 00:00:00+00:00 | 581311.0 | |
2023-12-31 00:00:00+00:00 | 522461.0 |
23 rows × 1 columns
Rolling/Window#
DataFrame.rolling(window, min_periods=None, center=False, win_type=None, on=None, axis=0, closed=None, step=None, method=’single’)
Shorthand methods:
cumsum()
pct_change()
rolling_df = test_df.pivot(index="datetime", columns="asset", values=test_metrics)
rolling_df
AdrActCnt | PriceUSD | |||
---|---|---|---|---|
asset | btc | eth | btc | eth |
datetime | ||||
2010-07-18 00:00:00+00:00 | 860.0 | NaN | 0.085840 | NaN |
2010-07-19 00:00:00+00:00 | 929.0 | NaN | 0.080800 | NaN |
2010-07-20 00:00:00+00:00 | 936.0 | NaN | 0.074736 | NaN |
2010-07-21 00:00:00+00:00 | 784.0 | NaN | 0.079193 | NaN |
2010-07-22 00:00:00+00:00 | 594.0 | NaN | 0.058470 | NaN |
... | ... | ... | ... | ... |
2023-02-03 00:00:00+00:00 | 988867.0 | 457502.0 | 23445.813177 | 1665.605250 |
2023-02-04 00:00:00+00:00 | 961115.0 | 500596.0 | 23355.524386 | 1671.046952 |
2023-02-05 00:00:00+00:00 | 802171.0 | 537666.0 | 22957.225191 | 1631.121142 |
2023-02-06 00:00:00+00:00 | 920525.0 | 503556.0 | 22745.165037 | 1614.274822 |
2023-02-07 00:00:00+00:00 | 944049.0 | 477572.0 | 23266.269204 | 1672.722311 |
4588 rows × 4 columns
rolling_df.rolling(5, min_periods=3, center=False).mean()
AdrActCnt | PriceUSD | |||
---|---|---|---|---|
asset | btc | eth | btc | eth |
datetime | ||||
2010-07-18 00:00:00+00:00 | NaN | NaN | NaN | NaN |
2010-07-19 00:00:00+00:00 | NaN | NaN | NaN | NaN |
2010-07-20 00:00:00+00:00 | 908.333333 | NaN | 0.080459 | NaN |
2010-07-21 00:00:00+00:00 | 877.250000 | NaN | 0.080142 | NaN |
2010-07-22 00:00:00+00:00 | 820.600000 | NaN | 0.075808 | NaN |
... | ... | ... | ... | ... |
2023-02-03 00:00:00+00:00 | 985010.400000 | 502227.2 | 23321.619961 | 1620.930898 |
2023-02-04 00:00:00+00:00 | 985760.800000 | 499124.4 | 23432.839386 | 1641.942616 |
2023-02-05 00:00:00+00:00 | 957325.400000 | 502775.8 | 23398.274041 | 1651.202627 |
2023-02-06 00:00:00+00:00 | 931324.800000 | 500124.8 | 23201.866527 | 1645.677170 |
2023-02-07 00:00:00+00:00 | 923345.400000 | 495378.4 | 23153.999399 | 1650.954095 |
4588 rows × 4 columns
Gaussian Window:
num = 18
std_dev = 4.5
window = scipy.signal.windows.gaussian(num, std=std_dev)
z_score = np.linspace(-num/2/std_dev, num/2/std_dev, num)
plt.plot(z_score, window)
plt.title(r"Gaussian window ($\sigma$=7)")
plt.ylabel("Amplitude")
plt.xlabel("Z-Score")
plt.figure()
<Figure size 640x480 with 0 Axes>
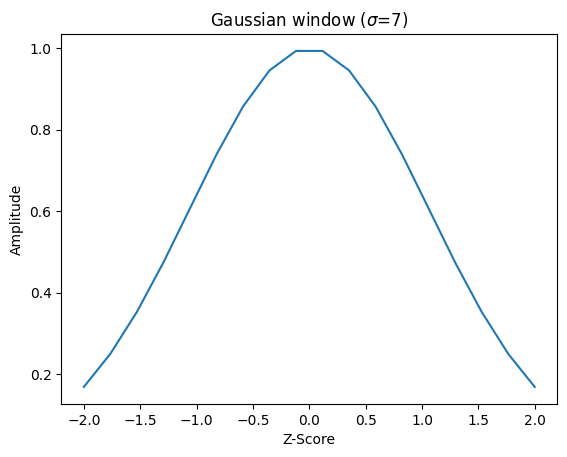
<Figure size 640x480 with 0 Axes>
Binning#
cut#
Bins are of equal size (width), but number of entries per bin may not similar.
# returns pd.Series
pd.cut(test_df['PriceUSD'], bins=5).value_counts()
(-67.491, 13508.392] 6459
(13508.392, 27016.733] 339
(40525.074, 54033.415] 211
(27016.733, 40525.074] 208
(54033.415, 67541.756] 112
Name: PriceUSD, dtype: int64
qcut#
Bins are not of equal size (width), but number of entries per bin are similar.
# returns pd.Series
pd.qcut(test_df['PriceUSD'], q=5).value_counts()
(0.0495, 16.825] 1466
(16.825, 294.001] 1466
(1095.26, 7548.085] 1466
(7548.085, 67541.756] 1466
(294.001, 1095.26] 1465
Name: PriceUSD, dtype: int64
Strings#
Regex cheat sheet here
Python string formatting cookbook
nba_df = pd.read_csv("C:/Users/wsaye/PycharmProjects/CashAppInterview/data/nba.csv")
nba_df.dropna(inplace=True)
nba_df
Name | Team | Number | Position | Age | Height | Weight | College | Salary | |
---|---|---|---|---|---|---|---|---|---|
0 | Avery Bradley | Boston Celtics | 0.0 | PG | 25.0 | 6-2 | 180.0 | Texas | 7730337.0 |
1 | Jae Crowder | Boston Celtics | 99.0 | SF | 25.0 | 6-6 | 235.0 | Marquette | 6796117.0 |
3 | R.J. Hunter | Boston Celtics | 28.0 | SG | 22.0 | 6-5 | 185.0 | Georgia State | 1148640.0 |
6 | Jordan Mickey | Boston Celtics | 55.0 | PF | 21.0 | 6-8 | 235.0 | LSU | 1170960.0 |
7 | Kelly Olynyk | Boston Celtics | 41.0 | C | 25.0 | 7-0 | 238.0 | Gonzaga | 2165160.0 |
... | ... | ... | ... | ... | ... | ... | ... | ... | ... |
449 | Rodney Hood | Utah Jazz | 5.0 | SG | 23.0 | 6-8 | 206.0 | Duke | 1348440.0 |
451 | Chris Johnson | Utah Jazz | 23.0 | SF | 26.0 | 6-6 | 206.0 | Dayton | 981348.0 |
452 | Trey Lyles | Utah Jazz | 41.0 | PF | 20.0 | 6-10 | 234.0 | Kentucky | 2239800.0 |
453 | Shelvin Mack | Utah Jazz | 8.0 | PG | 26.0 | 6-3 | 203.0 | Butler | 2433333.0 |
456 | Jeff Withey | Utah Jazz | 24.0 | C | 26.0 | 7-0 | 231.0 | Kansas | 947276.0 |
364 rows × 9 columns
Search/Replace#
# does not assume regex
# (nba_df["Name"].str.find("a") >= 1).sum()
nba_df["Name"].str.find("J") >= 1
0 False
1 False
3 True
6 False
7 False
...
449 False
451 True
452 False
453 False
456 False
Name: Name, Length: 364, dtype: bool
# assumes regex
# nba_df["Name"].str.contains("^[jJ]")
# nba_df["Name"].str.contains("^(Jo)")
nba_df["Name"].str.extract("^(Jo)")
0 | |
---|---|
0 | NaN |
1 | NaN |
3 | NaN |
6 | Jo |
7 | NaN |
... | ... |
449 | NaN |
451 | NaN |
452 | NaN |
453 | NaN |
456 | NaN |
364 rows × 1 columns
nba_df["Name"].str.replace("^(Jo)", "PORKY", regex=True)
0 Avery Bradley
1 Jae Crowder
3 R.J. Hunter
6 PORKYrdan Mickey
7 Kelly Olynyk
...
449 Rodney Hood
451 Chris Johnson
452 Trey Lyles
453 Shelvin Mack
456 Jeff Withey
Name: Name, Length: 364, dtype: object
Split, Concat#
# assumes regex if len > 1, else literal
nba_df["Name"].str.split(" ")
0 [Avery, Bradley]
1 [Jae, Crowder]
3 [R.J., Hunter]
6 [Jordan, Mickey]
7 [Kelly, Olynyk]
...
449 [Rodney, Hood]
451 [Chris, Johnson]
452 [Trey, Lyles]
453 [Shelvin, Mack]
456 [Jeff, Withey]
Name: Name, Length: 364, dtype: object
# assumes regex if len > 1, else literal
nba_df["Name"].str.split(" ", n=1, expand=True)
0 | 1 | |
---|---|---|
0 | Avery | Bradley |
1 | Jae | Crowder |
3 | R.J. | Hunter |
6 | Jordan | Mickey |
7 | Kelly | Olynyk |
... | ... | ... |
449 | Rodney | Hood |
451 | Chris | Johnson |
452 | Trey | Lyles |
453 | Shelvin | Mack |
456 | Jeff | Withey |
364 rows × 2 columns
nba_df["Name"].str.split(" ").str.len().describe()
count 364.000000
mean 2.032967
std 0.220220
min 2.000000
25% 2.000000
50% 2.000000
75% 2.000000
max 5.000000
Name: Name, dtype: float64
nba_df["Name"].str.cat(others=[nba_df["Team"], nba_df["College"]], sep="-")
0 Avery Bradley-Boston Celtics-Texas
1 Jae Crowder-Boston Celtics-Marquette
3 R.J. Hunter-Boston Celtics-Georgia State
6 Jordan Mickey-Boston Celtics-LSU
7 Kelly Olynyk-Boston Celtics-Gonzaga
...
449 Rodney Hood-Utah Jazz-Duke
451 Chris Johnson-Utah Jazz-Dayton
452 Trey Lyles-Utah Jazz-Kentucky
453 Shelvin Mack-Utah Jazz-Butler
456 Jeff Withey-Utah Jazz-Kansas
Name: Name, Length: 364, dtype: object
Formatting#
# nba_df["Name"].str.upper()
nba_df["Name"].str.lower()
0 avery bradley
1 jae crowder
3 r.j. hunter
6 jordan mickey
7 kelly olynyk
...
449 rodney hood
451 chris johnson
452 trey lyles
453 shelvin mack
456 jeff withey
Name: Name, Length: 364, dtype: object
nba_df['Salary_str'] = (nba_df['Salary']/10**6).map('${:,.2f}M'.format) # for single Series
nba_df['Salary_str']
# cohort_crosstab = cohort_crosstab.applymap('{:,.0f}%'.format) # for entire df
0 $7.73M
1 $6.80M
3 $1.15M
6 $1.17M
7 $2.17M
...
449 $1.35M
451 $0.98M
452 $2.24M
453 $2.43M
456 $0.95M
Name: Salary_str, Length: 364, dtype: object
Modeling#
Linear Regression#
lin_reg_df = pd.read_csv('C:/Users/wsaye/PycharmProjects/CashAppInterview/data/lin_reg_test_data.csv') # load data set
X = lin_reg_df.iloc[:, 0].values.reshape(-1, 1) # values converts it into a numpy array
Y = lin_reg_df.iloc[:, 1].values.reshape(-1, 1) # -1 means that calculate the dimension of rows, but have 1 column
COLOR = lin_reg_df.iloc[:, 2].values.reshape(-1, 1)
ohe = OneHotEncoder(sparse_output=False)
ohe_vals = ohe.fit_transform(COLOR)
X_mat = np.concatenate([X, ohe_vals], axis=1)
def regression_results(y_true, y_pred, lin_reg):
# Regression metrics
explained_variance = metrics.explained_variance_score(y_true, y_pred)
mean_absolute_error = metrics.mean_absolute_error(y_true, y_pred)
mse = metrics.mean_squared_error(y_true, y_pred)
mean_squared_log_error = metrics.mean_squared_log_error(y_true, y_pred)
median_absolute_error = metrics.median_absolute_error(y_true, y_pred)
r2 = metrics.r2_score(y_true, y_pred)
print('explained_variance: ', round(explained_variance,4))
print('mean_squared_log_error: ', round(mean_squared_log_error,4))
print('r^2: ', round(r2,4))
print('MAE: ', round(mean_absolute_error,4))
print('MSE: ', round(mse,4))
print('RMSE: ', round(np.sqrt(mse),4))
print(f"Coefficients: {lin_reg.coef_}")
print(f"Intercept: {lin_reg.intercept_}")
Test/Train Split#
X_train, X_test, y_train, y_test = train_test_split(X_mat, Y, test_size = 0.25)
Fit, Predict, Summarize#
regr = LinearRegression()
regr.fit(X_train, y_train)
y_test_pred = regr.predict(X_test)
lin_reg_df["y_pred"] = regr.predict(X_mat)
regression_results(y_test, y_test_pred, regr)
explained_variance: 0.955
mean_squared_log_error: 0.0135
r^2: 0.9548
MAE: 7.8925
MSE: 103.3243
RMSE: 10.1649
Coefficients: [[ 1.30312052 110.24915657 -120.19158265 9.94242608]]
Intercept: [-1.35003848]
K-Means#
from sklearn.cluster import KMeans
X_ktrain, X_ktest, y_ktrain, y_ktest = train_test_split(X, Y, test_size = 0.25)
train_set = np.concatenate([X_ktrain, y_ktrain], axis=1)
test_set = np.concatenate([X_ktest, y_ktest], axis=1)
kmeans = KMeans(n_clusters=3, random_state=0, n_init="auto").fit(train_set)
train_labels = kmeans.labels_
test_labels = kmeans.predict(test_set)
Plot Model:
fig = make_subplots(rows=1, cols=2)
fig.add_trace(go.Scatter(x=X_ktrain[:, 0], y=y_ktrain[:, 0], mode='markers', name='train',
marker=dict(color=train_labels, line_color="black", line_width=1)), row=1, col=1)
fig.add_trace(go.Scatter(x=X_ktest[:, 0], y=y_ktest[:, 0], mode='markers', name='test',
marker=dict(color=test_labels, line_color="black", line_width=1, symbol="x")), row=1, col=2)
fig.show()
Plotting#
Matplotlib#
# plt.scatter(X, Y)
# plt.plot(X, Y_pred, color="red", linestyle='None', marker="x")
plt.scatter(lin_reg_df["x"], lin_reg_df["y"])
plt.plot(lin_reg_df["x"], lin_reg_df["y_pred"], color="green", linestyle='None', marker="x")
plt.show()
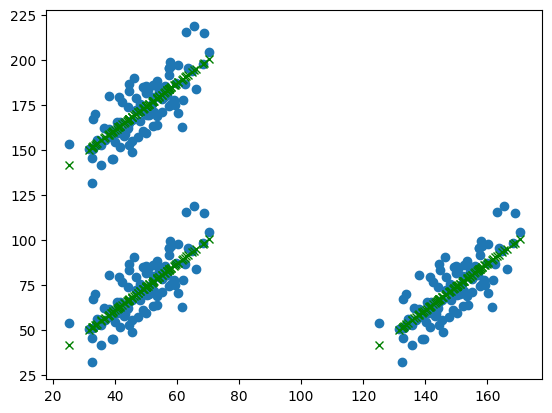
Plotly (Standard)#
fig = go.Figure()
fig.add_trace(go.Scatter(x=X[:, 0], y=Y[:, 0], mode='markers', name='raw data', marker=dict(color="grey")))
# fig.add_trace(go.Scatter(x=X[:, 0], y=Y_pred[:, 0], mode='markers', name='prediction', marker=dict(color=COLOR[:, 0])))
for c in list(np.unique(COLOR[:, 0])):
temp_x = lin_reg_df.loc[lin_reg_df["color"]==c, "x"]
temp_y = lin_reg_df.loc[lin_reg_df["color"]==c, "y_pred"]
fig.add_trace(go.Scatter(x=temp_x, y=temp_y, mode='lines', name='pred-' + c, line_color=c))
fig.show()
Plotly Express#
fig = px.scatter(lin_reg_df, x="x", y="y", color="color")
fig.show()
fig = px.scatter(lin_reg_df, x="x", y="y", color="color",
facet_col="color",
# facet_row="time",
# trendline="ols"
)
fig.show()
Sample Analyses#
Cohort#
rand_gen = np.random.RandomState(2021) # set seed
start_date = dt.datetime.strptime("2022-01-01", "%Y-%m-%d")
end_date = dt.datetime.strptime("2022-01-10", "%Y-%m-%d")
date_rng = pd.date_range(start_date, end_date).values
total_days = len(date_rng)
num_users = 1000
user_df_list = []
for u in range(0, num_users):
num_active_days = rand_gen.randint(low=2, high=total_days)
active_days_index = rand_gen.randint(low=0, high=total_days, size=(1, num_active_days))
active_dates = pd.Series(date_rng[active_days_index[0, :]])
user_id = pd.Series([u]*num_active_days)
user_df = pd.concat([active_dates, user_id], axis=1)
user_df.columns = ["date", "user_id"]
user_df_list.append(user_df)
cohort_df = pd.concat(user_df_list)
cohort_df
date | user_id | |
---|---|---|
0 | 2022-01-06 | 0 |
1 | 2022-01-10 | 0 |
2 | 2022-01-01 | 0 |
3 | 2022-01-07 | 0 |
4 | 2022-01-06 | 0 |
... | ... | ... |
2 | 2022-01-10 | 999 |
3 | 2022-01-06 | 999 |
4 | 2022-01-10 | 999 |
5 | 2022-01-03 | 999 |
6 | 2022-01-10 | 999 |
5443 rows × 2 columns
first_date = cohort_df.groupby(by=["user_id"]).min().rename(columns={"date": "start_date"})
cohort_df = cohort_df.join(first_date, on="user_id", how="left")
cohort_df
date | user_id | start_date | |
---|---|---|---|
0 | 2022-01-06 | 0 | 2022-01-01 |
1 | 2022-01-10 | 0 | 2022-01-01 |
2 | 2022-01-01 | 0 | 2022-01-01 |
3 | 2022-01-07 | 0 | 2022-01-01 |
4 | 2022-01-06 | 0 | 2022-01-01 |
... | ... | ... | ... |
2 | 2022-01-10 | 999 | 2022-01-03 |
3 | 2022-01-06 | 999 | 2022-01-03 |
4 | 2022-01-10 | 999 | 2022-01-03 |
5 | 2022-01-03 | 999 | 2022-01-03 |
6 | 2022-01-10 | 999 | 2022-01-03 |
5443 rows × 3 columns
cohort_crosstab = pd.crosstab(cohort_df['start_date'], cohort_df['date'])
cohort_totals = np.diag(cohort_crosstab).reshape(-1, 1)
cohort_crosstab[cohort_crosstab.columns] = 100 * cohort_crosstab.values / cohort_totals
cohort_crosstab = cohort_crosstab.applymap('{:,.0f}%'.format)
cohort_crosstab
date | 2022-01-01 | 2022-01-02 | 2022-01-03 | 2022-01-04 | 2022-01-05 | 2022-01-06 | 2022-01-07 | 2022-01-08 | 2022-01-09 | 2022-01-10 |
---|---|---|---|---|---|---|---|---|---|---|
start_date | ||||||||||
2022-01-01 | 100% | 39% | 45% | 43% | 42% | 45% | 47% | 47% | 34% | 42% |
2022-01-02 | 0% | 100% | 44% | 42% | 45% | 41% | 43% | 45% | 38% | 42% |
2022-01-03 | 0% | 0% | 100% | 46% | 32% | 39% | 31% | 42% | 37% | 40% |
2022-01-04 | 0% | 0% | 0% | 100% | 38% | 43% | 33% | 39% | 35% | 43% |
2022-01-05 | 0% | 0% | 0% | 0% | 100% | 42% | 38% | 64% | 38% | 39% |
2022-01-06 | 0% | 0% | 0% | 0% | 0% | 100% | 24% | 37% | 44% | 44% |
2022-01-07 | 0% | 0% | 0% | 0% | 0% | 0% | 100% | 47% | 63% | 42% |
2022-01-08 | 0% | 0% | 0% | 0% | 0% | 0% | 0% | 100% | 25% | 31% |
2022-01-09 | 0% | 0% | 0% | 0% | 0% | 0% | 0% | 0% | 100% | 167% |
2022-01-10 | 0% | 0% | 0% | 0% | 0% | 0% | 0% | 0% | 0% | 100% |
Funnel#
stages = ["Website visit", "Downloads", "Potential customers", "Requested price", "Invoice sent"]
df_mtl = pd.DataFrame(dict(number=[39, 27.4, 20.6, 11, 3], stage=stages))
df_mtl['office'] = 'Montreal'
df_toronto = pd.DataFrame(dict(number=[52, 36, 18, 14, 5], stage=stages))
df_toronto['office'] = 'Toronto'
df = pd.concat([df_mtl, df_toronto], axis=0)
fig = px.funnel(df, x='number', y='stage', color='office')
display(fig)
fig2 = make_subplots()
fig2.add_trace(go.Funnel(y = stages,
x = [49, 29, 26, 11, 2],
textinfo = "value+percent initial"))
fig2